A narrative review about regulatory acceptability standards for clinical assays
Introduction
Many clinical chemistry assays produce results that can be compared to a reference method procedure. This allows regulatory bodies such as Food and Drug Administration (FDA) to have acceptance protocols that require evaluation results between the candidate and reference assay to meet certain acceptability limits. Besides acceptability limits, most standards include a protocol, analysis, and reporting method. A generic 510(k) expectation from the FDA is that 95% of results are within limits (1). This is a total error specification, which as shown below can be estimated by various means. The 510(k) standard refers to the CLSI EP21 (Clinical Laboratory Standards Institute, Evaluation Protocol) total analytical error standard (2) as an approved standard but does not require its use.
The problem with this acceptability stratagem is that if 95% percent of results are within limits, then up to 5% of results can be outside of limits for an approved assay. If a result is far away from reference, then potentially serious patient harm exists. For example, if a glucose result is reported as 350 mg/dL when truth is 30 mg/dL, there is the danger that insulin will be given to an already hypoglycemic patient. If a blood lead assay reads 2.3 µg/dL when truth is 75 µg/dL, emergency treatment for high lead exposure may not be initiated due to a lead result below the cutoff limit.
This paper covers the problems arising from such acceptability standards including:
- A generic problem with the standards;
- Protocols used to evaluate the standards;
- How the data are analyzed;
- How often results are observed that potentially can cause serious patient harm;
- Why people do not pay more attention to dangerous results.
Note that this paper does not apply to standards that require 100% agreement such as the recent FDA standard for COVID-19 molecular assays (3). I present the following article in accordance with the Narrative Review reporting checklist (available at http://dx.doi.org/10.21037/jlpm-21-3).
A hierarchy among performance standards
This article deals mainly with regulatory performance standards. One can view performance standards as belonging to different types. In the US, regulatory performance standards from the FDA are at the top of the hierarchy because the performance standards must be met for assays to be sold. Standards such as EP21 (or other CLSI performance standards such as precision and linearity) are quasi regulatory standards. They are accepted by the FDA although their use is not a requirement. EP21 suggests the protocol and analysis for method comparison studies but does not proscribe acceptable limits. While ISO 15197 is not adopted by the FDA, it is routinely used in publications to demonstrate acceptable performance for glucose meters.
The ISO 15189 standard, based on ISO 9001, while largely an exercise in documentation does have a performance section as discussed below. It is in the process of becoming a regulatory standard by accreditation bodies such as CAP (College of American Pathologists).
The ISO 15189 standard contains a measurement uncertainty estimation requirement (4,5). Measurement uncertainty provides a 95% confidence interval for the measurement, which is of course different from requiring 95% of values to be contained by a set of limits. Whereas measurement uncertainty was originally estimated by an extremely complicated “bottoms-up” approach (6,7), a recently proposed “top-down” approach has been advocated (8,9). This top-down approach is a combination of measuring the precision of controls and assessing traceability. Unfortunately, it ignores any patient specific interference, user error, or other pre- and post-analytical error. A real top-down approach can be achieved using a fault tree and is as complicated as the bottoms-up approach (10).
Finally, there are performance standards which are largely academic as they have no regulatory status and do not inform whether as assay can be marketed. The most recent such performance standard is contained in an issue of Clinical Chemistry and Laboratory Medicine—the so-called Milan conference (11). A hierarchy for acceptable performance was given as (I) the effect of measurement performance on clinical outcomes; (III) the biological variation; (III) or state-of-the-art. The only example provided of measurement performance on clinical outcomes was a computer simulation. The glucose meter error grid was not mentioned even though it was prepared specifically to describe the effect of measurement performance on clinical outcomes.
The problem with the acceptability limits provided by regulatory standards
Providing one set of acceptance limits for 95% of the results implies that all results within limits are identical in not causing patient harm and that all results outside of limits are identical in causing patient harm. But if one has two results, one just inside and the other just outside limits, the two results have nearly the same error (12). For many diseases, it is illogical to think that one result will not cause harm and the other will. Moreover, as stated above, for many diseases, large errors cause much more patient harm than small errors. The concept of harm dependence on the difference from reference is exemplified in a glucose meter error grid and error grids have been proposed for all assays (13,14).
Providing a percentage of acceptable results is foreign to most specifications in medicine. One would never see a specification that surgeries should be conducted on the correct patient and organ 95% of the time.
The problem with the method comparison protocols
Quoting from Cuthbert Daniel (15) regarding an experiment, “the observations must be a fair (representative, random) sample of the population about which inferences are desired.” The main experiment to determine if a regulatory standard is met is the method comparison protocol and most protocols do not meet the above quoted criteria and are therefore biased.
For example, to randomly sample representative reagents would mean randomly selecting reagents from the population of reagents used throughout the lifetime of the assay. But for a newly developed assay, most reagents will exist only in the future—hence the reagents sampled are not random. This is an example of an unavoidable bias, but it is a bias nonetheless. An additional and avoidable bias is the removal of results from the method comparison. For example, EP21 Section 3.3 states that results should be discarded from errors due to a “wrong sample tested or a short sample, etc.” In the ISO 15197 standard for glucose meters (16), similar language is used whereby “If a measurement result is generated during a performance evaluation, it may be excluded from the data only in the following circumstances: —the blood-glucose monitoring system user recognizes that an error was made and documents the details.”
Thus, these protocols only evaluate the analytical error of the device and discard pre- and postanalytical errors. Now there is probably no clinical chemist who disagrees with eliminating a result caused by the wrong sample tested but consider the impact from a clinician’s standpoint.
Clinicians make decisions based in part on laboratory results and if a wrong sample tested in the method comparison protocol is representative of what would happen in routine testing, then an adequate assessment of potential patient harm can only be made if this error is retained (and this applies to all pre- and postanalytical errors).
Whereas these standards have a separate section devoted to user error, there is no attempt to consolidate the separate evaluations to arrive at overall acceptance criteria. And published evaluations cite only results from the analytical error evaluation as evidence of acceptability.
The problem with the analysis methods
A common analysis method to demonstrate that 95% of the results are within limits is to analyze method comparison data by regression to estimate average bias, perform a precision experiment to estimate precision and then to combine average bias and precision to estimate total error = average bias ± 2× the standard deviation.
There are several problems with this approach. A high and low outlier will make the average bias zero, but if the high and low outliers are representative, then they will occur, and the total estimation analysis will be incorrect. The problem with using multiples of precision is that it gives an impression that the spread of the data is governed by a normal distribution. As an example, consider a glucose meter value of 100 mg/dL (reference value also 100 mg/dL) with a 2.4%-meter coefficient of variation (CV). This implies that 95% of the glucose values will be no more than 4.8 mg/dL from reference, and well within the 15% limit required by ISO 15197 standard. A glucose value of 115 is 6.25 standard deviations away from 100 and would happen once every 5 billion results. Now if the reference value were 30 for the same glucose meter result (100 mg/dL with a 2.4% CV); this implies the 100 mg/dL result is 29 standard deviations away. This would occur once every 5×10187 samples. But the problem is that results outside of limits often come from different distributions. For example, a common glucose meter error (17) is user error caused by an inadequate sample. These same problems apply to the popular Six Sigma method. In Six Sigma, a unitless number is calculated as [total error limit − (average) assay bias]/standard deviation of assay. The above glucose meter gives Six Sigma =6.25. Hence, this would be a highly desirable assay although dangerous results could still be present since user errors and interferences are not included in Six Sigma.
The analysis method in the CLSI total error standard EP21 is a nonparametric method of estimating the central 95% of results. The nonparametric method was chosen to not have to worry about non normal data. This is an improvement, yet the limits reported still comprise only 95% of the results.
Whether a parametric or non-parametric is used to estimate the central 95% of data, virtually no analysis methods attempt to estimate the frequency of large errors. Each result in a method comparison can be considered to either yield a large error or not to yield a large error (18). For any sample size in the method comparison, one can estimate the 95% confidence interval for the frequency of large errors. For example, if the sample size in a method comparison is 300 and no large errors were observed, one can be 95% confident that no more than 1% of results contain large errors (19). The reality is that evaluations experiments are poor in proving that rare events do not occur.
The frequency of harmful results
Since up to 5% unacceptable results are allowed one can ask how many large errors actually occur? In the US, the FDA has a database of adverse events (20) commonly called MAUDE (Manufacturer and User Facility Device Experience). Unfortunately, useful rate information (number of events/usage) is often not available because usage figures of interest (such as by brand or time) are unavailable. Nevertheless, the rates must be exceedingly small due to the large number of observations. For example, the number of adverse events in 2020 for continuous glucose monitors (CGM) was 228,073 (21). The number of annual CGM results in the US is estimated as 233.4 billion (12/hour × 24 × 365 × 30% CGM users of 7.4 million insulin users) which gives a rate of 9.8E−07. Yet, if one constructs a rate based on (number of events/people that use CGM), rates are much larger. For example, in 2020, based on 30% CGM users of the 7.4 million people with diabetes that use insulin (=2.2 million), the rate of CGM adverse events is 10.3%. Another way of looking at the data is that in 2020, there were 625 CGM adverse events each day. Thus, the percentage of adverse events is well below the allowed 5%, but the percentage of people that have adverse events is alarming.
Other assays also appear in MAUDE. For example, in 2019, Table 1 shows the most frequent adverse events classified by the MAUDE term GENERIC_NAME. Of course, not all events are reported.
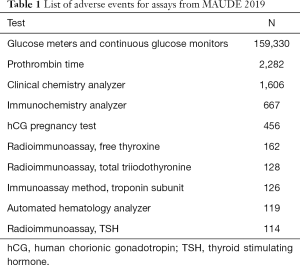
Full table
The problem when assays fail acceptance criteria
For any assay that fails the acceptance criteria, the logical conclusion is that the corresponding regulatory body should not allow that assay to be used (or to remove an assay that is in use). However, if an assay is not allowed to be used, the benefit of not having wrong results must be weighed against the harm caused by the lack of information from the assay. For example, consider a new glucose meter that is by far less expensive than any other meter but has failed the ISO 15197 acceptance standard. If a cohort of people with diabetes was not using glucose meters due to cost, yet wanted to use the failed meter, they would likely be better off using the failed meter than not using any meter.
The psychology of acceptability
An assay deemed acceptable has had it results reviewed by a regulatory body often based on a standard that has been prepared by a panel of experts. Companies market these assays not as having met a standard but rather having results that have exceeded the standard. For an assay judged to be acceptable, one does not expect unacceptable results and more importantly, one is unlikely to question the results. An example of this occurred at the University of Washington where a patient was treated for suspected trophoblastic carcinoma including a hysterectomy and the partial removal of one lung, based on 45 elevated human chorionic gonadotropin (hCG) tests. But finally, by performing a different hCG assay on the sample, it was determined that all the previous hCG tests were false positives—the patient never had cancer (22). Nor was this an isolated instance (23).
Why people do not pay more attention to dangerous results
No one wants to see dangerous results and there is no magic bullet to prevent them. Yet, subtle factors exist. Since acceptable assays according to regulatory standards require 95% of results within limits, there is less focus on the very small rate of unacceptable results that may occur. When such results are found to be caused by instrument or reagent problems, companies will feverishly work to solve those problems. However, as mentioned for glucose meters, many problems are caused by user error and this error source receives less focus (in adverse event reporting, user error must be reported as an adverse event for the device being used). And publications using adverse events as a data source are rare. Some unacceptable results are caused by interferences and it is difficult to evaluate every possible candidate interfering substance. Reliability tools such as Failure Mode Effects Analysis (FMEA), fault trees and Failure Reporting And Corrective Action System (FRACAS) (24-26) help prevent errors but they are not employed as vigorously as in other industries such as aerospace and automotive. Finally, there is a long history of evaluating only the analytical performance of assays.
Recommendations
Specifications should better reflect the harm when the magnitude of error increases. This could be achieved by using error grids instead of a single acceptance limit.
Despite the problems with method comparison protocol and analyses, they still provide valuable information because one wants to know the location of most results from reference. Total error for 95% of results should be calculated nonparametrically as described in EP21. Most protocols provide analytical performance only (without pre- and post-analytical error). However, results should never be discarded. The performance of an assay under ideal conditions and stripped of pre- and post-analytical error is misleading. Hence, results should be provided with and without pre- and post-analytical error. For assays on the market, the MAUDE database should be examined for adverse events.
More focus is needed on tools that prevent large errors, especially if pre- and post-analytical errors are detected. These include improved user training, FMEA, fault trees and FRACAS.
Conclusions
There will always be standards with limits for acceptable results. But we need to add tools to these standards that minimize the probability of large errors.
Acknowledgments
Funding: None.
Footnote
Reporting Checklist: The author has completed the Narrative Review reporting checklist. Available at http://dx.doi.org/10.21037/jlpm-21-3
Peer Review File: Available at http://dx.doi.org/10.21037/jlpm-21-3
Conflicts of Interest: The author has completed the ICMJE uniform disclosure form (available at http://dx.doi.org/10.21037/jlpm-21-3). The author has no conflicts of interest to declare.
Ethical Statement: The author is accountable for all aspects of the work in ensuring that questions related to the accuracy or integrity of any part of the work are appropriately investigated and resolved.
Open Access Statement: This is an Open Access article distributed in accordance with the Creative Commons Attribution-NonCommercial-NoDerivs 4.0 International License (CC BY-NC-ND 4.0), which permits the non-commercial replication and distribution of the article with the strict proviso that no changes or edits are made and the original work is properly cited (including links to both the formal publication through the relevant DOI and the license). See: https://creativecommons.org/licenses/by-nc-nd/4.0/.
References
- Recommendations for Dual 510(k) and CLIA Waiver by Application Studies Guidance for Industry and Food and Drug Administration Staff Document issued on February 26, 2020. Available online: https://www.fda.gov/media/109574/download Accessed December 9
- EP21 Evaluation of Total Analytical Error for Quantitative Medical Laboratory Measurement Procedures, 2nd Edition. Clinical and Laboratory Standards Institute PO Box 633 Annapolis Junction, MD 20701 USA.
- Policy for Coronavirus Disease-2019 Tests During the Public Health Emergency (Revised) Immediately in Effect Guidance for Clinical Laboratories, Commercial Manufacturers, and Food and Drug Administration Staff Document issued on the web on May 11, 2020. Accessed December 9, 2010. Available online: https://www.fda.gov/media/135659/download
- College of American Pathologists Measurement Uncertainty Guide - ISO 15189 Accreditation Program (cap.org). Available online: https://documents.cap.org/documents/cap15189-accreditation-program-measurement-uncertainty-guide.pdf
- Sciacovelli L, Aita A, Padoan A, et al. ISO 15189 accreditation and competence: a new opportunity for laboratory medicine. J Lab Precis Med 2017;2:79. [Crossref]
- Krouwer JS. Critique of the Guide to the expression of uncertainty in measurement method of estimating and reporting uncertainty in diagnostic assays. Clin Chem 2003;49:1818-21. [Crossref] [PubMed]
- Kristiansen J. The Guide to expression of uncertainty in measurement approach for estimating uncertainty: an appraisal. Clin Chem 2003;49:1822-9. [Crossref] [PubMed]
- ISO/TS 20914:2019 Medical laboratories — Practical guidance for the estimation of measurement uncertainty. Available online: https://www.iso.org/standard/69445.html
- Braga F, Panteghini M. The utility of measurement uncertainty in medical laboratories. Clin Chem Lab Med 2020;58:1407-13. [Crossref] [PubMed]
- Fault tree handbook. Systems and Reliability Research Office of Nuclear Regulatory Research. Washington, D.C. 20555: U.S. Nuclear Regulatory Commission. Available online: https://www.nrc.gov/docs/ML1007/ML100780465.pdf
- Panteghini M, Ceriotti F, Jones G, et al. Strategies to define performance specifications in laboratory medicine: 3 years on from the Milan Strategic Conference. Clin Chem Lab Med 2017;55:1849-56. [Crossref] [PubMed]
- Krouwer JS, Cembrowski GS. A review of standards and statistics used to describe blood glucose monitor performance. J Diabetes Sci Technol 2010;4:75-83. [Crossref] [PubMed]
- Parkes JL, Slatin SL, Pardo S, et al. A new consensus error grid to evaluate the clinical significance of inaccuracies in the measurement of blood glucose. Diabetes Care 2000;23:1143-8. [Crossref] [PubMed]
- Krouwer JS, Cembrowski GS. Towards more complete specifications for acceptable analytical performance - a plea for error grid analysis. Clin Chem Lab Med 2011;49:1127-30. [Crossref] [PubMed]
- Daniel C. Applications of statistics to industrial experimentation. New York, NY: Wiley, 1976.
- International Organization for Standardization. In vitro diagnostic test systems—requirements for blood-glucose monitoring systems for self-testing in managing diabetes mellitus. ISO 15197:2013. Available online: https://www.iso.org/standard/54976.html
- Corl D, Yin T, Ulibarri M, et al. What Can We Learn From Point-of-Care Blood Glucose Values Deleted and Repeated by Nurses? J Diabetes Sci Technol 2018;12:985-91. [Crossref] [PubMed]
- Krouwer JS. Recommendation to treat continuous variable errors like attribute errors. Clin Chem Lab Med 2006;44:797-8. [Crossref] [PubMed]
- Hahn GJ, Meeker WQ. Statistical intervals. A guide for practitioners. New York: Wiley, 1991:104.
- FDA MAUDE. Manufacturer and User Facility Device Experience. Accessed December 9, 2020. Available online: https://www.accessdata.fda.gov/scripts/cdrh/cfdocs/cfmaude/search.cfm
- Krouwer JS. Adverse Event Data for Years 2018 to 2020 for Diabetes Devices. J Diabetes Sci Technol 2021; [Crossref] [PubMed]
- Sainato D. How labs can minimize the risks of false positive results. Clin Lab News 2001;27:6-8.
- Rotmensch S, Cole LA. False diagnosis and needless therapy of presumed malignant disease in women with false-positive human chorionic gonadotropin concentrations. Lancet 2000;355:712-5. Erratum in: Lancet 2000 Aug 12;356(9229):600. [Crossref] [PubMed]
- FMEA. Accessed December 9, 2020. Available online: https://asq.org/quality-resources/fmea
- FRACAS. Accessed December 9, 2020. Available online: https://en.wikipedia.org/wiki/Failure_reporting,_analysis,_and_corrective_action_system
- Lim CY, Loh TP, Badrick T. Asking why: moving beyond error detection to failure mode and effects analysis. J Lab Precis Med 2020;5:29. [Crossref]
Cite this article as: Krouwer JS. A narrative review about regulatory acceptability standards for clinical assays. J Lab Precis Med 2021;6:18.