The added prognostic role of the hemoglobin, albumin, lymphocyte, and platelet scores in glioblastoma
Highlight box
Key findings
• The hemoglobin, albumin, lymphocyte, and platelet (HALP) score improved prognostication performance in glioblastoma (GBM) patients.
What is known and what is new?
• The area under the receiver operating characteristic curve (AUC) for prognostication ranged from 0.629 to 0.776 according to the Cox-based nomogram from prior studies.
• The present study found that the patients with high HALP a significantly longer survival time than patients with low HALP.
• Additionally, AUC of the nomogram using HALP score was 0.778, while AUC of the nomogram without HALP score was 0.710.
What is the implication, and what should change now?
• The HALP score was effective for improved prognostication in patients with GBM. A nomogram containing basic predictors could be utilized in allocating funding for costly standard chemotherapy in the limited-resource setting.
Introduction
Glioblastoma (GBM) is the most common malignant tumor in adults, as well as the most aggressive primary brain tumor (1,2). However, this tumor has the poorest prognosis among gliomas. Following tumor removal and adjuvant chemoradiotherapy, the median survival time for GBM has been reported to be between 11 and 15 months (1-3). Although targeted therapy has been studied for extending survival time, its application in real-world settings is limited by its high cost (4,5). One of the key methods for allocating resources is disease prognostication. Treatment plans that depend on a disease’s prognosis being positive present a management problem (6,7). The Karnofsky performance status (KPS), the extent of resection, and concurrent chemoradiotherapy with temozolomide (TMZ), various molecular biomarkers have all been investigated and reported as being linked with the prognosis in prior studies (7-10).
Several immune-nutritional indexes have recently been studied and reported as prognostic factors in a variety of cancers (11,12). Njoku et al. reported preoperative hemoglobin, albumin, lymphocyte, and platelet (HALP) scores and prognostic nutritional index (PNI) significantly associated with prognosis in endometrial cancer (13) and Leetanaporn et al. found that lower HALP score was related to improved survival time in patients with locally advanced cervical cancer (14). However, Shen et al. found that HALP scores had no predictive value in small-cell lung cancer and PNI was not significantly associated with recurrence-free survival in gastric cancer (15). Moreover, the neutrophil-to-lymphocyte ratio (NLR) is one of the immune-inflammatory biomarkers that has been studied as a prognostic factor. Garrett et al. showed that NLR and PNI were significantly connected to prognosis in univariate analysis; however, these biomarkers were not significantly associated with survival in multivariable analysis (16). Various immune-nutritional indexes associated with long survival time that are potentially explained by the indexes can represent patient status both nutritional and inflammatory status and reflect the severity of the disease (17,18).
Nomograms are frequently used in prognosis prediction because they reduce the complexity of the statistical risk predictive model into numerical estimates for death, recurrence, or other clinical outcomes (19). Li et al. developed a nomogram for predicting the overall prognosis of GBM patients using various clinical parameters and reported that the concordance indices (C-indices) of the prediction tool ranged from 0.729 to 0.734, whereas Kudulaiti et al. created a Cox-based nomogram and reported C-indices for prognostication ranging from 0.629 to 0.776 (20,21). Because patients with GBM had a poor prognosis, prognostication with high-precision predictive performance has been challenged.
A review of the literature indicates that there hasn’t been much research on the predictive value of immune-nutritional indices for GBM patients. Additionally, the immune-nutritional-based prediction model may improve the predictive performance of GBM patients’ prognosis. Faced with this gap, the authors aimed to evaluate the prognostication of HALP score, PNI, and NLR in patients with GBM. Additionally, the secondary objective study was to compare how well clinical nomograms with and without immune-nutritional indexes for 2-year mortality prediction could be performed in the future. We present this article in accordance with the TRIPOD reporting checklist (available at https://jlpm.amegroups.org/article/view/10.21037/jlpm-23-66/rc).
Methods
Study designs and study population
The present study was a retrospective cohort design. Between January 2000 and December 2021, all patients newly diagnosed with GBM were operated on at the tertiary center of southern Thailand. Patients who lacked complete medical records, or histological slides for diagnosis confirmation, or were unable to determine an updated status were excluded. GBM was diagnosed by pathologists with histological findings in microvascular proliferation or necrosis or wild-type IDH, according to the World Health Organization’s central nervous system tumor classification. In addition, the area under the receiver operating characteristic (ROC) curve (AUC) formula was used for sample size calculation (22). Based on Li et al., various parameters were calculated as follows: AUC of 0.729, alpha of 0.05, and estimation error of 0.10 (20). Therefore, the sample size of the study population was at least 108 patients.
Before the review of the medical records, the operational definition was made. Based on the research conducted by Bloch et al., the extent of resection and a residual tumor was assessed using post-operative T1-weighted (T1W) with contrast imaging. In detail, a residual tumor of less than 5% after surgery was referred to as total resection, but a leftover tumor of 5% or more was referred to as partial resection. Furthermore, a biopsy just served as a diagnostic tool; the tumor was not intended to be removed (23).
The formula for calculating the HALP score is [hemoglobin (g/L) × albumin (g/L) × lymphocytes (/L)]/platelets (/L) (23), while PNI was computed as follows: [albumin (g/L) + 5 × total lymphocyte count (109/L)] (24).
Ethical considerations
The study was conducted in accordance with the Declaration of Helsinki (as revised in 2013). The present study was approved by the Human Research Ethics Committee of the Faculty of Medicine, Prince of Songkla University (REC. 63-372-10-1). Because this study utilized a retrospective study design, informed consent from patients was not required. However, patient identification numbers were encoded before analysis.
Statistical analysis
Patients’ characteristics, neuroimaging findings, and treatment were demonstrated in proportion for descriptive purposes. When the variables were continuous, the mean with standard deviation (SD) was used. The updated patients’ status was classified as binary classifiers (death or living status) for the independent variable. In the present study, the complete case strategy was employed for missing value management before analyzing the final model. Therefore, the chi-square test was used to estimate the difference in each variable’s distribution across datasets, while the independent t-test was used to compare the mean of continuous variables across datasets. P values less than 0.05 were considered statistically significant.
In the survival analysis, overall survival, survival probability, and median survival time were evaluated. The Kaplan-Meier curves illustrate the overall survival, which is the period from surgery to death, and the survival probability was defined as the proportion of units that survive beyond a specified time such as a 2-year survival probability. In addition, the median survival time was defined as the length of time since surgery that half of a group of GBM patients were still living (25). The survival outcome of the present study was classified as either death or living status, which was confirmed on February 2, 2023, by the local civil registry database.
Nomogram development
A 70:30 split was used to randomly separate the entire data set into train and test datasets. The nomogram was trained using 70% of the total data, with the remaining 30% utilized to validate the prediction of the model. In detail, Cox proportional hazard regression was used to investigate factors associated with survival outcomes. Therefore, the candidate variables that had a P value of less than 0.10 in the univariate analysis were also included in the multivariable analysis. Therefore, backward stepwise selection with the lowest Akaike information criterion was performed for the final predictive model.
Nomogram was built from the final prediction model and the discriminative ability of a final model is measured by Harrell’s C-index. For internal validation, the bootstrap sampling method was used 1,000 times.
Hence, a prognosis of a 2-year mortality nomogram was estimated with unseen data from the remaining test dataset. To demonstrate the performance of the model on the test data set for which the actual values of the outcome were known, a confusion matrix was constructed as follows: sensitivity, specificity, positive predictive value (PPV), and negative predictive value (NPV). The ROC curve and the AUC were also utilized to evaluate the model’s discrimination. AUC of 0.7 would typically indicate acceptable discrimination, whereas AUCs of 0.8 and 0.9 would indicate good and excellent discrimination, respectively (26,27). The statistical analysis and nomogram development were performed using the R version 4.2.2 software (R Foundation, Vienna, Austria). Additionally, the nomogram in the present study was built by package ‘regplot’ (28).
Results
Clinical and radiological characteristics
Initially, 288 patients were diagnosed with GBM for the first time. Ten patients lacked a histopathological-confirmed diagnosis, five lacked complete information from their medical records, and two lacked preoperative radiological imaging. Therefore, 271 GBM patients were included in the total dataset. Hence, one hundred ninety patients were randomly assigned to the training dataset, which included the remaining 81 patients.
From the total dataset, the average age was 51.62 years (SD: 16.02 years) and the male-to-female ratio was 1.42. Furthermore, it was noted that more than half had preoperative KPS lower than 80. According to the imaging findings, the frontal and temporal lobes were the most common sites of GBM involvement, accounting for 32.1% and 29.2%, respectively. GBM of the corpus callosum was found in 10.3% of all patients in the present study and the average tumor size was 5.13 cm (SD: 1.80 cm). Moreover, 17% of this cohort had multiple tumors. Following the operation, total tumor resection was achieved in 22.9% of cases, while partial resection was performed in 63.8%. Consequently, postoperative KPS lower than 80 was found in 60.1%, and postoperative TMZ with radiotherapy was accomplished in 31.7% of cases. The majority of GBM (91.7%) were IDH-wildtype tumors, with IDH-mutant GBM occurring in 8.3% of cases. In the immune-nutritional indexes, the average HALP score, PNI, and NLP were 40.28 (SD: 25.75), 93.53 (SD: 48.32), and 7.04 (SD: 9.57), respectively.
After random splitting, the baseline characteristics, and preoperative laboratories according to the train and test datasets were shown in Tables 1,2. Almost all clinical characteristics were not substantially different across the train and test datasets, except for gender, preoperative ataxia, average maximum tumor diameter, and white cell count. However, these characteristics were not candidate variables in the multivariable analysis.
Table 1
Factors | Total dataset (n=271) | Train dataset (n=190) | Test dataset (n=81) | P value |
---|---|---|---|---|
Age (years), n (%) | 0.56 | |||
<60 | 184 (67.9) | 127 (66.8) | 57 (70.4) | |
≥60 | 87 (32.1) | 63 (33.2) | 24 (29.6) | |
Age (years), mean (SD) | 51.62 (16.02) | 50.84 (16.27) | 53.43 (15.38) | 0.21 |
Gender, n (%) | 0.001 | |||
Male | 159 (58.7) | 123 (64.7) | 36 (43.8) | |
Female | 112 (41.3) | 67 (35.3) | 45 (56.3) | |
Clinical presentation, n (%) | ||||
Progressive headache | 139 (51.3) | 99 (52.1) | 40 (49.4) | 0.75 |
Motor weakness | 129 (47.6) | 91 (47.9) | 38 (46.9) | 0.95 |
Seizure | 62 (22.9) | 45 (23.7) | 17 (21.0) | 0.66 |
Cranial nerve palsy | 33 (12.2) | 25 (13.2) | 8 (9.9) | 0.87 |
Behavior change | 24 (8.9) | 14 (7.4) | 10 (12.3) | 0.18 |
Ataxia | 6 (2.2) | 4 (2.1) | 2 (2.5) | 0.004 |
Preoperative KPS, n (%) | 0.77 | |||
<80 | 147 (54.2) | 102 (53.7) | 45 (55.6) | |
≥80 | 124 (45.8) | 88 (46.3) | 36 (44.4) | |
Major tumor location, n (%) | ||||
Frontal lobe | 87 (32.1) | 56 (29.5) | 31 (38.3) | 0.15 |
Temporal lobe | 79 (29.2) | 59 (31.1) | 20 (24.7) | 0.29 |
Parietal lobe | 50 (18.5) | 32 (16.8) | 18 (22.2) | 0.29 |
Corpus callosum | 28 (10.3) | 20 (10.5) | 8 (9.9) | 0.87 |
Occipital lobe | 13 (4.8) | 10 (5.3) | 3 (3.7) | 0.58 |
Periventricular region | 8 (3.0) | 6 (3.2) | 2 (2.5) | 0.75 |
Brainstem | 6 (2.2) | 3 (1.6) | 3 (3.7) | 0.27 |
Intraventricular region | 4 (1.5) | 2 (1.1) | 2 (2.5) | 0.37 |
Cerebellum | 2 (0.7) | 1 (0.5) | 1 (1.2) | 0.53 |
Pineal region | 2 (0.7) | 2 (1.1) | 0 (0.0) | 0.35 |
Basal ganglion or thalamic region | 14 (5.1) | 12 (6.3) | 2 (2.5) | 0.19 |
Multiple tumor, n (%) | 46 (17.0) | 33 (17.4) | 13 (16.0) | 0.79 |
Lateralization, n (%) | 0.41 | |||
Left | 120 (44.3) | 80 (42.1) | 40 (49.4) | |
Right | 118 (43.5) | 83 (43.7) | 35 (43.2) | |
Midline | 22 (8.1) | 20 (10.5) | 2 (2.5) | |
Bilateral | 11 (4.1) | 7 (3.7) | 4 (4.9) | |
Tumor diameter (cm), mean (SD) | 5.13 (1.80) | 5.28 (1.82) | 4.77 (1.71) | 0.03 |
Maximum diameter (cm), n (%) | 0.27 | |||
<5 | 120 (44.3) | 80 (42.1) | 40 (49.4) | |
≥5 | 151 (55.7) | 110 (57.9) | 41 (50.6) | |
Extent of resection, n (%) | 0.82 | |||
Biopsy | 36 (13.3) | 24 (12.6) | 12 (14.8) | |
Partial resection | 173 (63.8) | 121 (63.7) | 52 (64.2) | |
Total resection | 62 (22.9) | 45 (23.7) | 17 (21.0) | |
Postoperative KPS, n (%) | 0.46 | |||
<80 | 163 (60.1) | 117 (61.6) | 46 (56.8) | |
≥80 | 108 (39.9) | 73 (38.4) | 35 (43.2) | |
Adjuvant therapy, n (%) | 0.34 | |||
Radiotherapy alone | 185 (68.3) | 133 (70.0) | 52 (64.2) | |
Concomitant chemoradiotherapy (TMZ) | 86 (31.7) | 57 (30.0) | 29 (35.8) | |
IDH wild-type†, n (%) | 122 (91.7) | 119 (92.2) | 3 (75.0) | 0.92 |
†, the number of patients in the total dataset, train dataset, and test dataset are 133, 129, and 4, respectively. SD, standard deviation; KPS, Karnofsky performance status; TMZ, temozolomide.
Table 2
Factors | Total dataset | Train dataset | Test dataset | P value |
---|---|---|---|---|
Hematocrit (%), mean (SD) | 40.57 (4.69) | 40.68 (4.81) | 40.33 (4.42) | 0.57 |
Hemoglobin (g/dL), mean (SD) | 13.5 (1.66) | 13.63 (1.66) | 13.44 (1.66) | 0.39 |
White cell count (×103/mcL), mean (SD) | 10,987.90 (4,148.97) | 11,367.10 (4,250.60) | 10,103.09 (3,780.30) | 0.02 |
Neutrophil (%), mean (SD) | 73.75 (13.78) | 74.64 (13.53) | 71.68 (11.15) | 0.25 |
Lymphocyte (%), mean (SD) | 19.13 (10.77) | 18.62 (10.59) | 20.29 (11.15) | 0.24 |
Platelet count (×103/mcL), mean (SD) | 270,525.93 (86,802.07) | 276,522.81 (88,381.82) | 256,530.86 (81,823.57) | 0.08 |
Albumin (g/dL), mean (SD) | 3.95 (0.55) | 3.91 (0.58) | 4.05 (0.49) | 0.06 |
NLR, mean (SD) | 7.04 (9.57) | 7.17 (8.78) | 6.72 (11.25) | 0.74 |
PNI, mean (SD) | 93.53 (48.32) | 95.28 (51.78) | 89.45 (39.07) | 0.31 |
HALP score, mean (SD) | 40.28 (25.75) | 39.85 (16.27) | 41.29 (23.51) | 0.65 |
NLR, neutrophil-to-lymphocyte ratio; PNI, prognostic nutritional index; HALP, hemoglobin, albumin, lymphocyte, and platelet; SD, standard deviation.
Survival analysis
From the total dataset, 1-, 2-, and 5-year survival probabilities were 34% [95% confidence interval (CI): 28–40%], 14% (95% CI: 10–19%), and 3.4% (95% CI: 1.7–7.1%) and the average follow-up time was 14.4 months (SD: 16.4 months). Moreover, the median overall survival time was 8 months (95% CI: 7–10), as shown in Figure 1A. There were no significant differences in the survival curves of the train and test datasets (log-rank test, P value =0.13), as shown in Figure 1B.
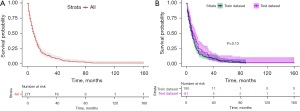
The Cox proportional hazard regression was performed to explore factors associated with prognosis. Age, preoperative KPS, basal ganglion or thalamic GBM, multiple GBM, the extent of resection, TMZ, postoperative KPS, PNI, and HALP score were candidate variables in univariate analysis, as shown in Figure 2. Thus, these candidates were employed to evaluate in multivariable analysis using a backward stepwise procedure. The final predictive model comprised age, preoperative KPS, basal ganglion or thalamic GBM, multiple GBM, the extent of resection, TMZ, and HALP score, as shown in Table 3.
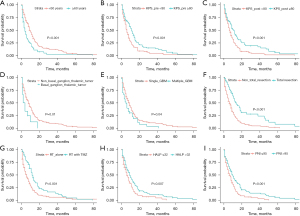
Table 3
Factors | Univariate analysis | Multivariable analysis | |||
---|---|---|---|---|---|
Hazard ratio (95% CI) | P value | Hazard ratio (95% CI) | P value | ||
Age (years) | 1.01 (1.007–1.027) | 0.001 | 1.01 (1.007–1.031) | <0.001 | |
Gender | |||||
Male | Ref. | ||||
Female | 0.79 (0.57–1.09) | 0.16 | |||
Clinical presentation | |||||
Progressive headache† | 0.87 (0.64–1.18) | 0.39 | |||
Motor weakness† | 1.22 (0.90–1.66) | 0.18 | |||
Seizure† | 1.09 (0.77–1.56) | 0.60 | |||
Cranial nerve palsy† | 0.82 (0.53–1.26) | 0.38 | |||
Behavior change† | 0.62 (0.37–1.14) | 0.12 | |||
Ataxia† | 1.17 (0.27–12.67) | 0.56 | |||
Preoperative KPS | |||||
<80 | Ref. | Ref. | |||
≥80 | 0.60 (0.44–0.83) | 0.002 | 0.74 (0.53–1.04) | 0.09 | |
Major tumor location | |||||
Frontal lobe† | 0.80 (0.57–1.12) | 0.21 | |||
Temporal lobe† | 1.06 (0.76–1.47) | 0.72 | |||
Parietal lobe† | 1.21 (0.81–1.80) | 0.33 | |||
Corpus callosum† | 1.16 (0.72–1.88) | 0.52 | |||
Occipital lobe† | 0.91 (0.44–1.86) | 0.80 | |||
Periventricular region† | 0.71 (0.28–1.78) | 0.47 | |||
Brainstem† | 0.73 (0.13–5.29) | 0.76 | |||
Intraventricular region† | 3.24 (0.79–13.28) | 0.11 | |||
Cerebellum† | 1.62 (0.22–11.64) | 0.68 | |||
Pineal region† | 1.44 (0.65–5.83) | 0.60 | |||
Basal ganglion or thalamic region† | 2.02 (1.09–3.75) | 0.02 | 2.13 (1.12–4.04) | 0.02 | |
Multiple tumor† | 1.50 (1.01–2.22) | 0.04 | 1.46 (0.94–2.25) | 0.08 | |
Lateralization | |||||
Left | Ref. | ||||
Right | 0.97 (0.70–1.35) | 0.98 | |||
Midline | 2.13 (1.01–4.48) | 0.04 | |||
Bilateral | 1.12 (0.65–1.94) | 0.67 | |||
Maximum diameter (cm) | |||||
<5 | Ref. | ||||
≥5 | 1.005 (0.73–1.37) | 0.97 | |||
Extent of resection | |||||
Non-total resection | Ref. | Ref. | |||
Total resection | 0.45(0.39–0.67) | <0.001 | 0.53 (0.35–0.79) | 0.002 | |
Postoperative KPS | |||||
<80 | Ref. | ||||
>80 | 0.59 (0.43–0.82) | 0.002 | |||
Adjuvant therapy | |||||
Radiotherapy alone | Ref. | Ref. | |||
Concomitant chemoradiotherapy (TMZ) | 0.58 (0.41–0.81) | 0.002 | 0.48 (0.34–0.70) | <0.001 | |
IDH mutation | |||||
Mutant | Ref. | ||||
Wild-type | 1.05 (0.46–2.34) | 0.89 | |||
NLR | |||||
≤7 | Ref. | ||||
>7 | 1.33 (0.96–1.83) | 0.08 | |||
PNI | |||||
≤95 | Ref. | ||||
>95 | 0.62 (0.42–0.85) | 0.003 | |||
HALP score | |||||
≤32 | Ref. | Ref. | |||
>32 | 0.66 (0.48–0.89) | 0.007 | 0.65 (0.47–0.90) | 0.01 |
†, data showed only the “yes group”, while reference groups (no group) were hidden. GBM, glioblastoma; CI, confidence interval; ref., reference; KPS, Karnofsky performance status; TMZ, temozolomide; NLR, neutrophil-to-lymphocyte ratio; PNI, prognostic nutritional index; HALP, hemoglobin, albumin, lymphocyte, and platelet.
Comparison prognostic performances of nomograms without/with HALP score
A nomogram was built from the final predictive models with and without the HALP score, as shown in Figure 3A,3B. For the goodness of fit measure of the prediction models, Harrell’s C-index of the nomogram without the HALP score was 0.704, while the nomogram with the HALP score had an unadjusted C-index of 0.707. Figure 3C,3D shows that the calibration plots demonstrated that the nomograms with HALP score were better at predicting the chance of survival than the nomograms without HALP. The internal validation was performed with the bootstrap method. As a result, nomograms without and with HALP had bootstrap-corrected C-indices of 0.692 and 0.704, respectively.
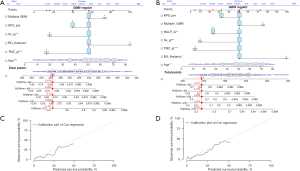
For 2-year death prediction with the testing dataset, the AUC of the nomogram without and with HALP was 0.710 and 0.778, as shown in Figure 4. Therefore, Table 4 demonstrates prognostic performances of 2-year mortality by nomogram without and with HALP score. The PPV of the nomogram without the HALP score was 0.86 (95% CI: 0.78–0.94), while the PPV of the nomogram with the HALP score increased to 0.95 (95% CI: 0.89–1.00).
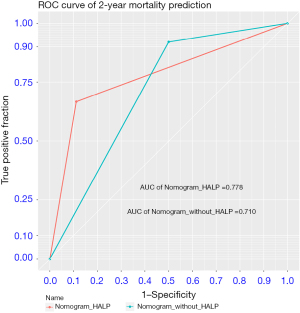
Table 4
Nomogram | Sensitivity (95% CI) | Specificity (95% CI) | PPV (95% CI) | NPV (95% CI) | AUC (95% CI) |
---|---|---|---|---|---|
Nomogram without HALP score | 0.92 (0.85–0.98) | 0.50 (0.26–0.73) | 0.86 (0.78–0.94) | 0.64 (0.39–0.89) | 0.71 (0.55–0.86) |
Nomogram with HALP score | 0.66 (0.55–0.78) | 0.88 (0.74–0.99) | 0.95 (0.89–1.00) | 0.43 (0.27–0.59) | 0.78 (0.66–0.89) |
HALP, hemoglobin, albumin, lymphocyte, and platelet; CI, confidence interval; PPV, positive predictive value; NPV, negative predictive value; AUC, area under the ROC curve; ROC, receiver operating characteristic.
Discussion
The present study found that the prognosis of GBM was poor, which was consistent with prior studies. Prognostic factors of the present study comprised age, basal ganglion/thalamic GBM, the extent of resection, TMZ, and HALP score these results are in concordance with other research reports. According to the literature review, the patient’s overall survival ranged from 8 to 16 months (1,29,30). The extent of resection, adjuvant chemoradiation with TMZ, and molecular biomarkers were all linked to a prolonged survival time in individuals with GBM (31,32).
As a result, the HALP score is one of the prognostic factors following multivariable analysis. This could be explained by the fact that the score evaluates both the patient’s immune system and nutritional state. According to Wang et al., the HALP cutoff value for endometrial cancer was 33.8, and found that the low-HALP group had poorer survival outcomes than the high-HALP group. Moreover, HALP was a prognostic factor with a cut-off value of 31.5 in patients with gastrointestinal stromal tumors (33). According to a review of the literature, few papers mention using the HALP score in GBM patients, and there is no standard cutoff point. As a result, the HALP cutoff in the present study was 32, and the high-HALP group had a significantly longer survival time than the low-HALP group. HALP score is significantly associated with favorable prognosis, which is probably explained by this parameter that is able to indicate patients’ systemic status, both nutritional and inflammatory status (17). In the present study, the predictive model with HALP had a high specificity that preferred a few false positives; thus, this predictive model was appropriate for confirming the occurrence of 2-year mortality (34). In addition, PNI has been studied as a prognostic factor in various cancers (26,35). PNI tended to be a predictor in this investigation since this biomarker was statistically significant in univariate analysis. However, PNI was not significantly associated with prognosis when the multivariable analysis was performed in the present study. In the future, a multicenter study or systematic review with meta-analysis may confirm the effect of PNI on prognosis from the increased sample size.
TMZ became the standard treatment following the study of Stupp’s trial. Nevertheless, the high expense of the standard treatment would be a financial burden in a limited-resource setting. Several studies proposed clinical prediction tools for prognostication in GBM. The HALP score may be used as a reliable and cheap prognostic factor for developing treatment strategies for GBM patients. Luo et al. developed a nomogram predicting prognosis in GBM from the SEER database and reported that the C-indices of the nomogram were 0.717 (95% CI: 0.710–0.724) and 0.724 (95% CI: 0.713–0.735) in the training cohort and the validation cohort, respectively (36). Additionally, Zheng et al. demonstrated the unadjusted C-index of a nomogram predicting prognosis in patients with GBM was 0.79, and the bootstrap-corrected C-index was 0.78, which is consistent with our findings (37). The nomogram with HALP score in the present study had a high value of PPV for 2-year mortality prediction; therefore, this tool with simple predictors could be an alternate way for allocating resources for high-cost medications in the real-world setting.
To the best of the authors’ knowledge, this was the first study to show the HALP-based nomogram for GBM prognosis. However, the limitations of the present study were noted. Firstly, the authors considered a small number of patients for the training and test datasets. This limitation would be overcome by multicenter research or meta-analysis, which would increase the number of study populations (38). Moreover, the nomogram should be estimated using a larger number of unseen data to confirm generalizability, which would make it challenging to evaluate these models in the future through a prospective study (2). Secondly, the retrospective design of the study led to the selection and information bias. Nevertheless, the authors tried to manage this limitation by establishing an operational definition, inclusion, and exclusion criteria before the review (39,40).
Conclusions
In summary, the HALP score was useful for the added performance of prognostication in patients with GBM. A nomogram with basic predictors could be challenged to apply for allocating resources for high-cost drugs. Furthermore, future multicenter prospective studies should be done to externally validate the HALP-based nomogram.
Acknowledgments
Funding: None.
Footnote
Reporting Checklist: The authors have completed the TRIPOD reporting checklist. Available at https://jlpm.amegroups.org/article/view/10.21037/jlpm-23-66/rc
Data Sharing Statement: Available at https://jlpm.amegroups.org/article/view/10.21037/jlpm-23-66/dss
Peer Review File: Available at https://jlpm.amegroups.org/article/view/10.21037/jlpm-23-66/prf
Conflicts of Interest: Both authors have completed the ICMJE uniform disclosure form (available at https://jlpm.amegroups.org/article/view/10.21037/jlpm-23-66/coif). The authors have no conflicts of interest to declare.
Ethical Statement: The authors are accountable for all aspects of the work in ensuring that questions related to the accuracy or integrity of any part of the work are appropriately investigated and resolved. The study was conducted in accordance with the Declaration of Helsinki (as revised in 2013). The present study was approved by the Human Research Ethics Committee of Faculty of Medicine, Prince of Songkla University (REC. 63-372-10-1). Because this study utilized a retrospective study design, informed consent from patients was not required. However, patient identification numbers were encoded before analysis.
Open Access Statement: This is an Open Access article distributed in accordance with the Creative Commons Attribution-NonCommercial-NoDerivs 4.0 International License (CC BY-NC-ND 4.0), which permits the non-commercial replication and distribution of the article with the strict proviso that no changes or edits are made and the original work is properly cited (including links to both the formal publication through the relevant DOI and the license). See: https://creativecommons.org/licenses/by-nc-nd/4.0/.
References
- Stupp R, Mason WP, van den Bent MJ, et al. Radiotherapy plus concomitant and adjuvant temozolomide for glioblastoma. N Engl J Med 2005;352:987-96. [Crossref] [PubMed]
- Tunthanathip T, Sangkhathat S. Temozolomide for patients with wild-type isocitrate dehydrogenase (IDH) 1 glioblastoma using propensity score matching. Clin Neurol Neurosurg 2020;191:105712. [Crossref] [PubMed]
- Stupp R, Hegi ME, Mason WP, et al. Effects of radiotherapy with concomitant and adjuvant temozolomide versus radiotherapy alone on survival in glioblastoma in a randomised phase III study: 5-year analysis of the EORTC-NCIC trial. Lancet Oncol 2009;10:459-66. [Crossref] [PubMed]
- Le Rhun E, Preusser M, Roth P, et al. Molecular targeted therapy of glioblastoma. Cancer Treat Rev 2019;80:101896. [Crossref] [PubMed]
- Lamers LM, Stupp R, van den Bent MJ, et al. Cost-effectiveness of temozolomide for the treatment of newly diagnosed glioblastoma multiforme: a report from the EORTC 26981/22981 NCI-C CE3 Intergroup Study. Cancer 2008;112:1337-44. [Crossref] [PubMed]
- Tunthanathip T, Sangkhathat S, Tanvejsilp P, et al. Prognostic Impact of the Combination of MGMT Methylation and TERT Promoter Mutation in Glioblastoma. J Neurosci Rural Pract 2021;12:694-703. [Crossref] [PubMed]
- Tunthanathip T, Sae-Heng S, Oearsakul T, et al. Quality of life, out-of-pocket expenditures, and indirect costs among patients with the central nervous system tumors in Thailand. J Neurosci Rural Pract 2022;13:740-9. [Crossref] [PubMed]
- Liang J, Lv X, Lu C, et al. Prognostic factors of patients with Gliomas - an analysis on 335 patients with Glioblastoma and other forms of Gliomas. BMC Cancer 2020;20:35. [Crossref] [PubMed]
- Hegi ME, Diserens AC, Gorlia T, et al. MGMT gene silencing and benefit from temozolomide in glioblastoma. N Engl J Med 2005;352:997-1003. [Crossref] [PubMed]
- Chen K, Shi Y, Luo W, et al. SMIM20: a new biological signal associated with the prognosis of glioblastoma. Transl Cancer Res 2023;12:2754-63. [Crossref] [PubMed]
- Farag CM, Antar R, Akosman S, et al. What is hemoglobin, albumin, lymphocyte, platelet (HALP) score? A comprehensive literature review of HALP's prognostic ability in different cancer types. Oncotarget 2023;14:153-72. [Crossref] [PubMed]
- Zhong H, Wang Y, Jia J, et al. Ferroptosis related genes are regulated by methylation and predict the prognosis of glioblastoma patients. Transl Cancer Res 2022;11:603-14. [Crossref] [PubMed]
- Njoku K, Barr CE, Ramchander NC, et al. Impact of pre-treatment prognostic nutritional index and the haemoglobin, albumin, lymphocyte and platelet (HALP) score on endometrial cancer survival: A prospective database analysis. PLoS One 2022;17:e0272232. [Crossref] [PubMed]
- Leetanaporn K, Hanprasertpong J. Predictive Value of the Hemoglobin-Albumin-Lymphocyte-Platelet (HALP) Index on the Oncological Outcomes of Locally Advanced Cervical Cancer Patients. Cancer Manag Res 2022;14:1961-72. [Crossref] [PubMed]
- Shen XB, Zhang YX, Wang W, et al. The Hemoglobin, Albumin, Lymphocyte, and Platelet (HALP) Score in Patients with Small Cell Lung Cancer Before First-Line Treatment with Etoposide and Progression-Free Survival. Med Sci Monit 2019;25:5630-9. [Crossref] [PubMed]
- Garrett C, Becker TM, Lynch D, et al. Comparison of neutrophil to lymphocyte ratio and prognostic nutritional index with other clinical and molecular biomarkers for prediction of glioblastoma multiforme outcome. PLoS One 2021;16:e0252614. [Crossref] [PubMed]
- Zhang D, Chen S, Cao W, et al. HALP score based on hemoglobin, albumin, lymphocyte and platelet can predict the prognosis of tongue squamous cell carcinoma patients. Heliyon 2023;9:e20126. [Crossref] [PubMed]
- Zhang B, Cheng Y, Li R, et al. Development of a novel angiogenesis-related lncRNA signature to predict the prognosis and immunotherapy of glioblastoma multiforme. Transl Cancer Res 2023;12:13-30. [Crossref] [PubMed]
- Tunthanathip T, Ratanalert S, Sae-Heng S, et al. Prognostic factors and clinical nomogram predicting survival in high-grade glioma. J Cancer Res Ther 2021;17:1052-8. [Crossref] [PubMed]
- Li H, He Y, Huang L, et al. The Nomogram Model Predicting Overall Survival and Guiding Clinical Decision in Patients With Glioblastoma Based on the SEER Database. Front Oncol 2020;10:1051. [Crossref] [PubMed]
- Kudulaiti N, Zhou Z, Luo C, et al. A nomogram for individualized prediction of overall survival in patients with newly diagnosed glioblastoma: a real-world retrospective cohort study. BMC Surg 2021;21:238. [Crossref] [PubMed]
- Thai Thanh Truc. Statistics and Sample Size Pro. 2020. [Cited 2023 Sep 2]. Available online: https://play.google.com/store/apps/details?id=thaithanhtruc.info.sass&hl=en_US
- Bloch O, Han SJ, Cha S, et al. Impact of extent of resection for recurrent glioblastoma on overall survival: clinical article. J Neurosurg 2012;117:1032-8. [Crossref] [PubMed]
- Chen XL, Xue L, Wang W, et al. Prognostic significance of the combination of preoperative hemoglobin, albumin, lymphocyte and platelet in patients with gastric carcinoma: a retrospective cohort study. Oncotarget 2015;6:41370-82. [Crossref] [PubMed]
- Tunthanathip T, Oearsakul T. Comparison of predicted survival curves and personalized prognosis among cox regression and machine learning approaches in glioblastoma. J Med Artif Intell 2023;6:10. [Crossref]
- Chen L, Bai P, Kong X, et al. Prognostic Nutritional Index (PNI) in Patients With Breast Cancer Treated With Neoadjuvant Chemotherapy as a Useful Prognostic Indicator. Front Cell Dev Biol 2021;9:656741. [Crossref] [PubMed]
- Jaruenpunyasak J, Duangsoithong R, Tunthanathip T. Deep learning for image classification between primary central nervous system lymphoma and glioblastoma in corpus callosal tumors. J Neurosci Rural Pract 2023;14:470-6. [Crossref] [PubMed]
- Marshall R. Package ‘regplot’. 2022 [Cited 2023 Mar 5]. Available online: https://cran.r-project.org/web/packages/regplot/regplot.pdf
- Marta GN, Moraes FY, Feher O, et al. Social determinants of health and survival on Brazilian patients with glioblastoma: a retrospective analysis of a large populational database. Lancet Reg Health Am 2021;4:100066. [Crossref] [PubMed]
- Tunthanathip T, Sangkhathat S, Tanvejsilp P, et al. The clinical characteristics and prognostic factors of multiple lesions in glioblastomas. Clin Neurol Neurosurg 2020;195:105891. [Crossref] [PubMed]
- Szylberg M, Sokal P, Śledzińska P, et al. MGMT Promoter Methylation as a Prognostic Factor in Primary Glioblastoma: A Single-Institution Observational Study. Biomedicines 2022;10:2030. [Crossref] [PubMed]
- Lacroix M, Abi-Said D, Fourney DR, et al. A multivariate analysis of 416 patients with glioblastoma multiforme: prognosis, extent of resection, and survival. J Neurosurg 2001;95:190-8. [Crossref] [PubMed]
- Wang J, Jiang P, Huang Y, et al. Prognostic Value of the Cutoffs for HALP in Endometrial Cancer. Am J Clin Oncol 2023;46:107-13. [Crossref] [PubMed]
- Supbumrung S, Kaewborisutsakul A, Tunthanathip T. Machine learning-based classification of pineal germinoma from magnetic resonance imaging. World Neurosurg X 2023;20:100231. [Crossref] [PubMed]
- Zhao Z, Yin XN, Wang J, et al. Prognostic significance of hemoglobin, albumin, lymphocyte, platelet in gastrointestinal stromal tumors: A propensity matched retrospective cohort study. World J Gastroenterol 2022;28:3476-87. [Crossref] [PubMed]
- Luo Y, Hao L, Liu C, et al. Prognostic model for predicting overall survival in patients with glioblastoma: an analysis based on the SEER database. J Investig Med 2023;71:439-47. [Crossref] [PubMed]
- Zheng L, Zhou ZR, Shi M, et al. Nomograms for predicting progression-free survival and overall survival after surgery and concurrent chemoradiotherapy for glioblastoma: a retrospective cohort study. Ann Transl Med 2021;9:571. [Crossref] [PubMed]
- Tunthanathip T. Malignant transformation in low-grade astrocytoma for long-term monitoring. J Cancer Res Ther 2022;18:1616-22. [Crossref] [PubMed]
- Kaewborisutsakul A, Tunthanathip T. Development and internal validation of a nomogram for predicting outcomes in children with traumatic subdural hematoma. Acute Crit Care 2022;37:429-37. [Crossref] [PubMed]
- Taweesomboonyat C, Kaewborisutsakul A, Tunthanathip T, et al. Necessity of in-hospital neurological observation for mild traumatic brain injury patients with negative computed tomography brain scans. J Health Sci Med Res 2020;38:267-74. [Crossref]
Cite this article as: Tunthanathip T, Oearsakul T. The added prognostic role of the hemoglobin, albumin, lymphocyte, and platelet scores in glioblastoma. J Lab Precis Med 2024;9:12.