Optimization and validation of moving average quality control for the INR and aPTT coagulation tests
Introduction
The analytical quality assurance of coagulation testing is complicated by the risk of applying non-commutable control materials and analytical drift related to reagent lot changes. Furthermore inherent to the practice of quality control measurements is its scheduled character. For all these reasons we aimed to optimize and validate moving average quality control (MA QC) procedures for the international normalized ratio (INR) and the activated partial thromboplastin time (aPTT) to enable real-time continuous quality control for these tests.
Recently there has been a renewed interest in the application of patient based real-time quality control and more specifically moving average quality control (MA QC) (1,2). This has been triggered by new methods to study and optimize MA QC and resulted in new insights in the understanding of MA QC (3-5). Furthermore, in todays practice of short test turnaround times and readily availability of lab results to support acute care and clinical care pathways, there is an increasing demand for continuous QC.
The newly developed approaches to study MA QC use simulation methods that aim to mimic the MA QC error detection in a realistic manner and generally use the results needed for error detection as readout (3,4). One of these methods is available in the online MA Generator application. This application uses a training set of historical laboratory test results to study laboratory specific MA QC performance. Bias detection curves are used to optimize MA QC and MA validation charts are used to obtain a more thorough insight in the MA QC performance of the selected optimal MA QC procedure. Recently we have described an approach to design quality control plans that integrate internal QC and MA QC (6). In this approach MA QC is studied when internal QC was considered to allow insufficient quality assurance; when no (stable) controls were available, tests were known for their rapid onset of critical error, non-commutability issues associated with the control materials or assays with low sigma values (≤4). This study focused on chemistry, immunochemistry and hemocytometry tests. As a follow-up we used the same approach to investigate the application of MA QC to support the analytical quality assurance of coagulation testing. In the Netherlands Cancer Institute a limited panel of coagulation tests are offered. Since only the international normalized ration (INR) and activated partial thromboplastin time (aPTT) tests fulfilled the recently postulated requirements to be studied for MA QC, our research focused on optimization and implementation of MA QC for these two coagulation tests. We present the following article in accordance with the MDAR Reporting Checklist (available at http://dx.doi.org/10.21037/jlpm-19-104).
Methods
INR and APTT Data collection
INR and aPTT results obtained from 1st January till the 30th of June 2019 were included in this study. INR and aPTT tests were run a Werfen ACL TOP 300 system. In our Cancer Clinic we do not have a thrombosis service and INR and aPTT tests are generally requested to support care of comorbidities, safely support (surgical) interventions and for emergency (bleeding) diagnostics. Data export was performed per test and according to the MA Generator requirements and contained the time of test results availability, analyzer identification and numerical value of the obtained result.
MA QC optimization
For MA QC optimization and validation the MA Generator application was used (www.huvaros.com, The Netherlands) (7). MA Generator incorporates the MA QC optimization method using bias detection curves and MA Validation charts as described by van Rossum and Kemperman (3,8). MA QC was investigated only for the mean calculation since our LIS (GLIMS, MIPS Gent Belgium) that is used for MA QC management only supports the mean calculation algorithm for MA QC. Next, bias detection curves were obtained and several variables for truncation limits (None, 1,5; 2,5 and 4.0 for INR and None, 50 and 100 for aPTT) and “window” (15, 20, 20, 40 and 50) were studied. Optimization focused on allowing most rapid detection of preferably all systematic errors. Therefore MA Generator was configured to study the error detection performance of systematic errors of −50%, −40%, −20%, −15%, −10%, −5%, −3%, −1%, 1%, 3%, 5%, 10%, 15%, 20%, 30%, 40%, and 50%. The performance of the selected optimal MA QC was presented in MA Validation charts.
Results
INR MA QC optimization and validation
A selection of obtained bias detection curves for the INR are presented in Figure 1. From these curves it was concluded that a truncation limits was required when using mean calculations and that a MA QC using a truncation limit of 2.5 and calculating the mean of the last 20 included patient results was considered optimal for our setting. Both the MA QC graph as well as the MA validation chart of the selected optimal MA QC procedure are presented in Figure 2.
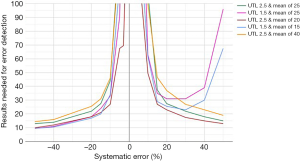
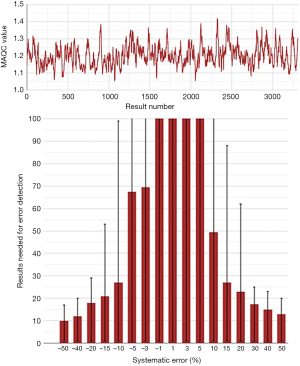
aPTT MA QC investigations
When studying MA QC for aPTT a shift in average aPTT values was noted (Figure 3A). When reviewing the data this was related to a reagent lot switch. At the time of this switch no significant change in quality control levels was noted (Figure 3B). For clearance of the new reagent lot a reagent comparison had been performed using 20 samples. Here an average difference of 1 second was observed which was considered acceptable. In the MA QC graph however the difference before and after the reagent switch seems to be significantly larger and the results obtained using the new reagent seem to be at least about 2 seconds higher. The 2 second shift was also on the longer term not clearly visible in the Levey-Jennings plot. Detection by internal QC was however potentially compromised by a change in QC materials as presented in the Levey-Jennings graph as presented by the new QC marks and alternative colored lines.
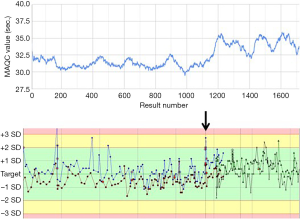
For the final optimization of the aPTT MA QC, only the results obtained after the reagent lot switch were used. A selection of the obtained bias detection curves are presented in Figure 4. The selected optimized aPTT MA QC is together with the MA Validation chart presented in Figure 5.
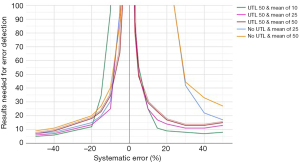
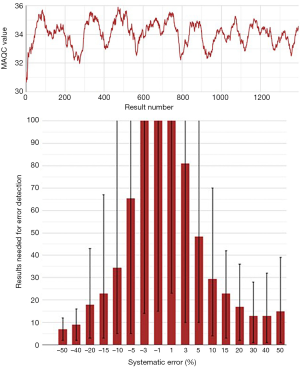
Discussion
In this study moving average quality control procedures for the INR and aPTT coagulation parameters are optimized to support the analytical quality assurance in an oncological hospital. For MA QC optimization, the online MA Generator application was used and optimization was based on recent INR and aPTT results obtained at the laboratory. Optimization included studying the MA QC patterns and comparison of MA QC error detection using bias detection curves. Finally MA validation charts of selected optimal MA QC procedures were obtained.
As for other laboratory specialties, also for coagulation testing proper quality assurance is of key importance. For coagulation diagnostics a large variation in internal QC practices have been identified but to our knowledge non used moving average or patient based real-time QC approaches to support analytical quality control (9). For several reasons MA QC might be an interesting option to include in QC plans of coagulation tests. The first is that for various coagulation tests, quality control material non-commutability is a relevant issue (10). By design MA QC has no commutability issue since it is based on true patient materials. Furthermore coagulation tests are known for its reagent lot to lot variations (11,12) and generally a more thorough verification procedure is required to allow acceptance and application of new reagent lots. To enable a more thorough and longitudinal insight in the effect of changing reagent lots MA QC might be of value. Based on these reasons we were interested in whether MA QC can support internal QC for quality assurance of the coagulation tests offered in our institute. Only the INR and aPTT were selected to be studied due to the low daily production of the coagulation tests available at our lab. For both, a MA QC would be desirable that enables real-time QC in between scheduled internal QC measurements as well as assuring longer-term test stability.
When studying MA QC for INR and APTT it was noted that for the aPTT MA QC a shift was observed in the training dataset of historical results. This shift was linked to a shift in reagent lot, an observation that was less clearly visible in the internal QC measurements. Furthermore a small reagent lot shift of about 1 second had been identified by the standard new reagent lot verification procedure. This was considered acceptable at that time. When reviewing the MA QC the shift seemed to be on average about 2 seconds with some variation, potentially also reflecting assay stability. Another complicating factor might have been that a little bit after the reagent lot shift new QC materials were applied complicating detection of analytical shifts. Alternatively the observed MA QC shift might reflect a change in patient populations for which an aPTT test was requested. However would have to coincide with the exact same time as the reagent lot change. The observed shift in MA QC, which did not trigger QC alarming and was not noticed by structural reviewing of the internal QC Levey-Jennings plot, illustrates that MA QC might support longer-term assay stability and is amongst others useful to detect shifts caused by reagent lot changes. Due to the shift in aPTT MA QC levels we choose to focus on the current MA QC level for further optimization and created a new aPTT MA Generator file consisting of only aPTT results obtained after the aPTT reagent lot shift.
Since our LIS supports only mean calculations we were forced to use truncation limits to enable relevant MA QC error detection for both aPTT and INR. The truncation limit selection was based on a trial and error approach using the bias detection curves as readout. Finally MA QC procedures were selected that in our view enabled the best combination of detecting positive as well as negative error and allowing both rapid detection of smaller systematic errors and detection of smaller systematic errors originating from longer-term analytical drift.
In conclusion the optimization and validation of MA QC is demonstrated for the aPTT and INR coagulation tests that resulted in relevant error detection performance. Furthermore an example of detecting relevant analytical drift by MA QC caused by a aPTT reagent lot change, not detected by alternative methods, is presented.
Acknowledgments
Funding: None.
Footnote
Provenance and Peer Review: This article was commissioned by the Guest Editor (Mark A. Cervinski) for the series “Patient Based Quality Control” published in Journal of Laboratory and Precision Medicine. The article has undergone external peer review.
Reporting Checklist: The authors have completed the MDAR reporting checklist. Available at http://dx.doi.org/10.21037/jlpm-19-104
Data Sharing Statement: Available at http://dx.doi.org/10.21037/jlpm-19-104
Conflicts of Interest: All authors have completed the ICMJE uniform disclosure form, available at http://dx.doi.org/10.21037/jlpm-19-104. The series “Patient Based Quality Control” was commissioned by the editorial office without any funding or sponsorship. Dr. van Rossum reports other from Huvaros, during the conduct of the study; and Dr. van Rossum is the founder, owner and director of Huvaros that markets the MA Generator used in this study to perform the simulation analysis, obtain the bias detection curves, MA Validation charts. The authors have no other conflicts of interest to declare.
Ethical Statement: The authors are accountable for all aspects of the work in ensuring that questions related to the accuracy or integrity of any part of the work are appropriately investigated and resolved.
Open Access Statement: This is an Open Access article distributed in accordance with the Creative Commons Attribution-NonCommercial-NoDerivs 4.0 International License (CC BY-NC-ND 4.0), which permits the non-commercial replication and distribution of the article with the strict proviso that no changes or edits are made and the original work is properly cited (including links to both the formal publication through the relevant DOI and the license). See: https://creativecommons.org/licenses/by-nc-nd/4.0/.
References
- van Rossum HH. Moving average quality control: Principles, practical application and future perspectives. Clin Chem Lab Med 2019;57:773-82. [Crossref] [PubMed]
- Badrick T, Bietenbeck A, Cervinski MA, et al. Patient-based real-time quality control: Review and recommendations. Clin Chem 2019;65:962-71. [Crossref] [PubMed]
- van Rossum HH, Kemperman H. Optimization and validation of moving average quality control procedures using bias detection curves and moving average validation charts. Clin Chem Lab Med 2017;55:218-24. [Crossref] [PubMed]
- Ng D, Polito FA, Cervinski MA. Optimization of a moving averages program using a simulated annealing algorithm: The goal is to monitor the process not the patients. Clin Chem 2016;62:1361-71. [Crossref] [PubMed]
- Fleming JK, Katayev A. Changing the paradigm of laboratory quality control through implementation of real-time test results monitoring: For patients by patients. Clin Biochem 2015;48:508-13. [Crossref] [PubMed]
- van Rossum HH, van den Broek D. Design and implementation of quality control plans that integrate moving average and internal quality control: Incorporating the best of both worlds. Clin Chem Lab Med 2019;57:1329-38. [Crossref] [PubMed]
- MA generator. Available online: www.huvaros.com (Accessed 2019).
- van Rossum HH, Kemperman H. A method for optimization and validation of moving average as continuous analytical quality control instrument demonstrated for creatinine. Clin Chim Acta 2016;457:1-7. [Crossref] [PubMed]
- McFarlane A, Aslan B, Raby A, Moffat KA, Selby R, Padmore R. Internal quality control practices in coagulation laboratories: Recommendations based on a patterns-of-practice survey. Int J Lab Hematol 2015;37:729-38. [Crossref] [PubMed]
- Jennings I, Kitchen D, Kitchen S, Woods T, Walker I. The importance of commutability in material used for quality control purposes. Int J Lab Hematol 2019;41:39-45. [Crossref] [PubMed]
- Rosborough TK. Comparing different lots of activated partial thromboplastin time reagent: Analysis of two methods. Am J Clin Pathol 1998;110:173-7. [Crossref] [PubMed]
- Findlater SH. Usefulness of cumulative summation of differences method for determining aptt reagent suitability. Clin Lab Sci 2012;25:142-8. [Crossref] [PubMed]
Cite this article as: van Rossum HH, Huijsman MN, Meeues C, van den Broek D. Optimization and validation of moving average quality control for the INR and aPTT coagulation tests. J Lab Precis Med 2020;5:27.